Ji Zhou, Robert Jackson and Felipe Pinheiro outline some of the ways the AI/Data Science team at NIAB is utilising AI to identify varieties based on photos as part of the One CGIAR Seed Equal Initiative.
Since our establishment in early 2020 the Data Sciences Department at NIAB has been using AI and machine learning techniques to advance multi-scale phenotyping and AI-powered trait analysis capabilities across NIAB and with academic and industrial collaborators.
For example, NIAB uses the drone-based aerial phenotyping platform, AirMeasurer, and IoT-based sensing (eg CropSight and CropQuant™) for monitoring agricultural and horticultural crops during the season. Equipment such as the Videometer and SeedGerm platforms are used to monitor seed quality, germination, and vigour testing.
Project funded by:
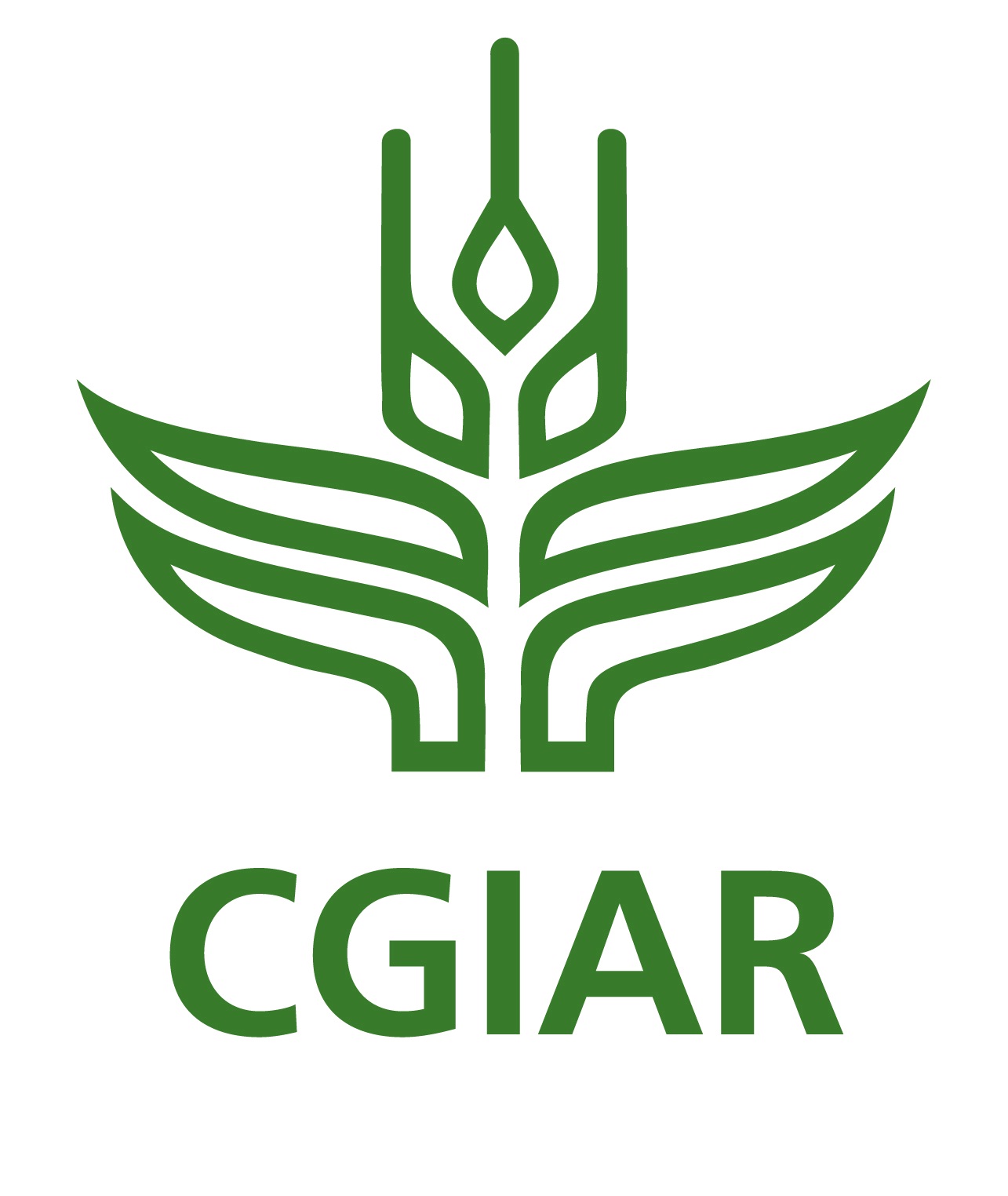
After a recent BBSRC award, NIAB jointly established a UK leading high-performance computing (HPC) and GPU clusters infrastructure with the James Hutton Institute, and other partners, for results dissemination, cloud-based informatics and AI predictive modelling.
With this background, One CGIAR asked us a simple question, can you build an AI method to identify specific food crop varieties from just a few pictures of the crop?
For context, One CGIAR is a global research partnership for a food secure future, dedicated to transforming food, land and water systems in a climate crisis through a $1 billion annual research portfolio.
The partnership unites 15 international organisations and has 10,000 staff working across 89 different countries, producing science that has brought benefits to hundreds of millions of people around the world. One CGIAR supports innovative solutions to improve food security, increase biodiversity, stimulate economic growth and strengthen resilience of farming systems.
Figure 2. Examples of ear count and yield prediction using the NIAB/Nanjing University YieldQuant-M app.
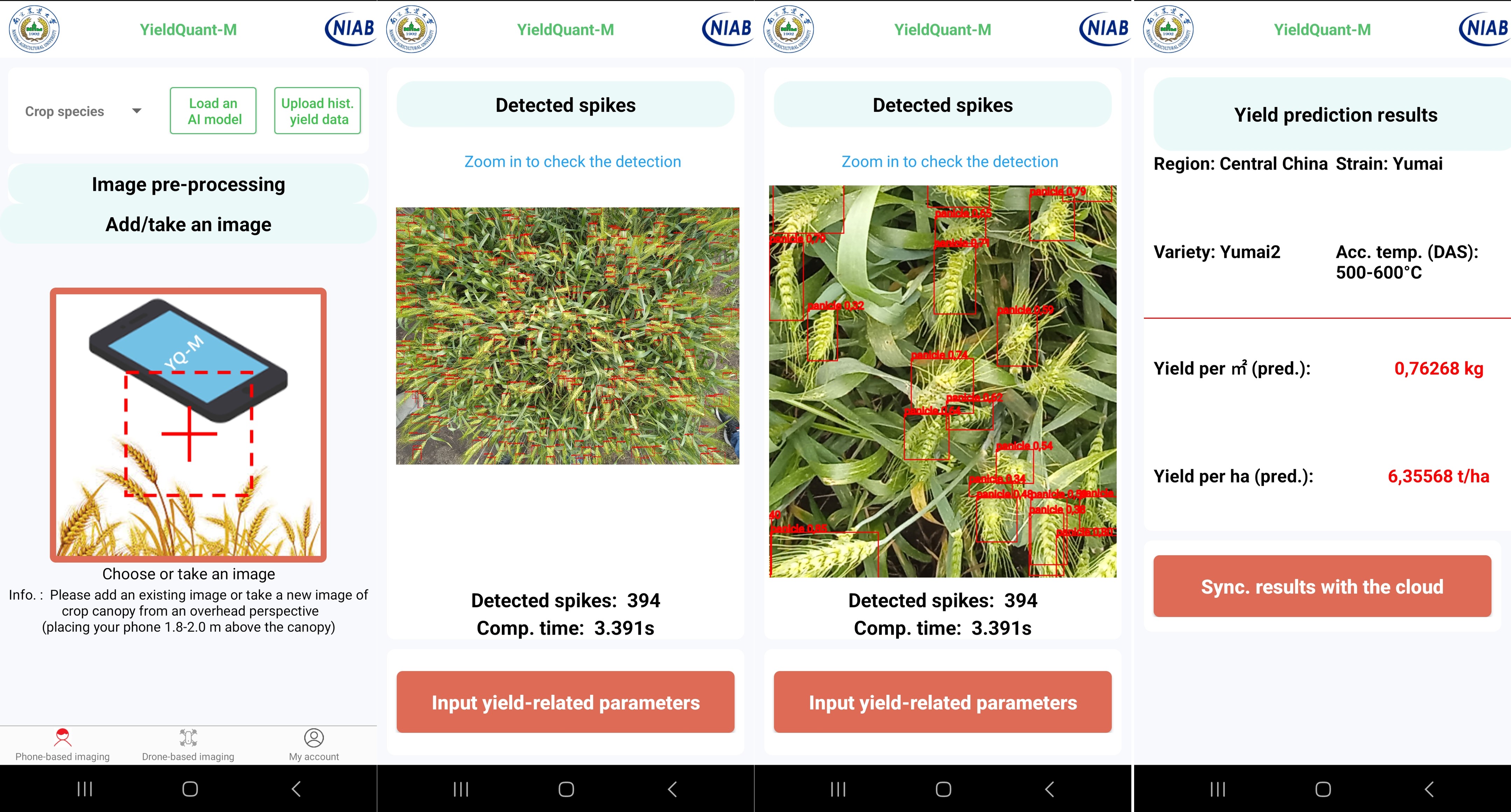
A key part of this is Seed Equal (Delivering Genetic Gains in Farmers’ Fields), which aims to support the equitable delivery of seed adapted to the various agronomic, climate and market challenges that farmers face. A fundamental of Seed Equal’s activities is to carry out AI-powered varietal identification.
Dr Ian Barker, a senior director for strategy delivery and scaling in the One CGIAR Genetic Innovation Science Area and is also based at NIAB in Cambridge, made the initial connection with Professor Zhou.
He is convinced by the power of AI for varietal identification: “I have observed potato experts at NIAB being trained in correctly identifying 200 different potato varieties. If humans can do this, AI can also do it this with our domain knowledge”.
This will also facilitate the accurate measurement of adoption rates of CGIAR varietal technologies and the prediction of yield to better measure genetic gain in farmer fields. And this is where the AI/Data Sciences team at NIAB comes in.
Figure 3. The VarScout app showing variety location in a region
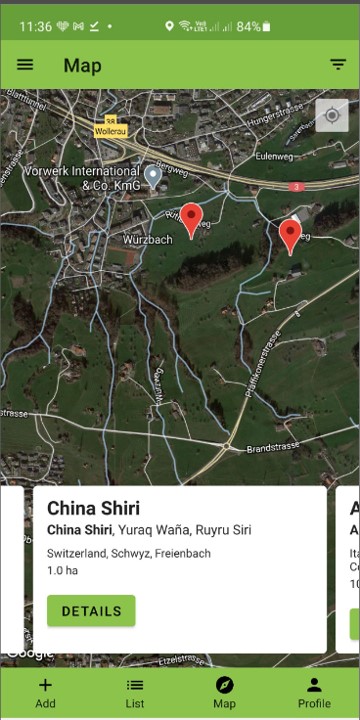
Through the awarded CGIAR initiative, we will contribute to “Combining cloud- and smartphone-based AI solutions for varietal identification of wheat and potato”, a project aims to advance the vision-based AI modelling tailored for varietal identification using cutting-edge AI algorithms such as Transformer and Visual Attention.
Currently, NIAB has pioneered AI-models to assess key yield components in wheat and are developing AI-based image recognition algorithms and open-source software (Figure 1).
With One CGIAR the ambition is to integrate this into VarScout, a digital ecosystem designed to record, store, and visualise crop varietal data on a local, national and international scale (Figure 2).
Dr Marcel Gatto, a senior scientist and agricultural economist at the International Potato Center and co-lead of this project highlighted that smallholder farmers in developing countries often do not know the identity of the varieties they are growing. Methods for accurate varietal identification exist, such as DNA fingerprinting, but are very costly and results are only available after months.
Powering VarScout with AI tools is a much-needed innovation to provide cost effective and real-time varietal adoption and yield data without compromising on data quality. This work has three main components:
- The development of AI-based models for cloud-based variety identification in wheat
- The integration of phone-based yield prediction and variety identification models into an Android application (APP) (Figure 3)
- The verification and improvement of the APP using One CGIAR’s resources for other food crops, such as potato, beans, etc.
To do this, first we needed to collect a set of images with which we could build a training set.
And so Felipe Pinheiro, from the Data Science group, packed his bags and flew off to Ethiopia. Ethiopia presents an interesting trial location for this work. Hosts of crop inspectors travel far and wide across the country to collect samples for DNA identification of different wheat varieties, and if they are collecting samples, they could also collect images? This would provide us with a dataset of variety images tied to known varieties.
Before image collection across the country could happen, Felipe spent a week training numerous groups of inspectors in the specific protocol for photographing wheat with camera phones provided by the One CGIAR team on the ground (Figure 4).
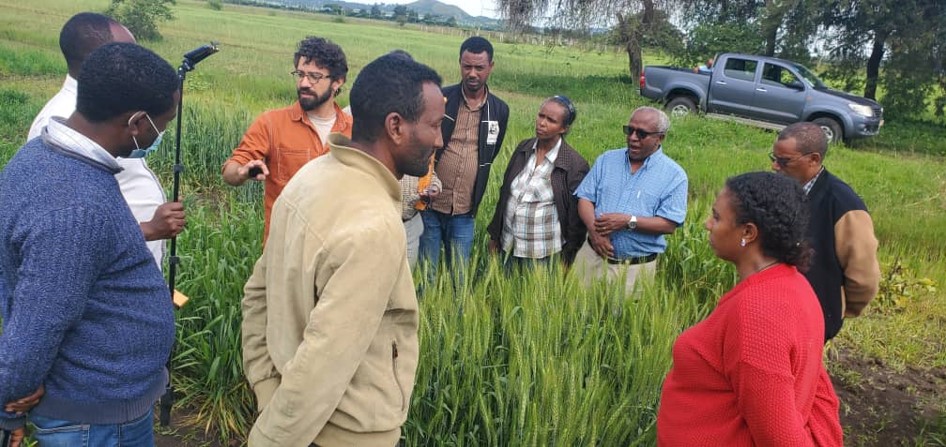
Figure 4. NIAB's Felipe Pinheiro in Ethiopia training crop inspectors in plot imaging
Whilst this sounds simple it is a key step. The success or failure of an AI prediction model regularly rests on the quality and number of images that will be used in training the AI models.
Another important success factor is to take the pictures from agronomic varietal trials where the 20 most dominant wheat varieties were planted as, on smallholder farms, wheat fields can be a mix of different varieties. Therefore, Felipe had to train 100+ inspectors in a new method of image collection, ensuring consistent and high-resolution images with right organ parts acquired.
Now completed, this dataset along with a similar one from the UK are being used to build a baseline AI model for key and variety identification.
This is where the cutting-edge AI aspect comes into the project with attention mechanisms (a process assigning different weights to different parts of the image based on the relevance to the task at hand) to identify defining organ parts, by which specific varieties can be identified from the high-level (colour and shape) and low-level (texture and pixel connections) features in the photographs. Once completed, the prototype will be tested in 2024 verses farmers’ responses and DNA fingerprinted data collected for the same varieties.
Finally, we will explore incorporating the app into the current VarScout app aiding farmers, agronomists, extension workers or anyone to record the location of where a particular crop variety is growing based solely on imagery.
So, how is AI helping farmers now?
Well, we are already using it in field mapping and grain assessment in breeding, and the next step for the AI/Data Science team and One CGIAR is phone-based variety identification and yield predictions allowing us to accurately monitor yield and measure genetic gains in farmers’ fields.
This article originally appeared in the Winter 2023/4 edition of NIAB’s Landmark magazine. Landmark features in-depth technical articles on all aspects of NIAB crop research, comment and advice. You can sign up for free and get Landmark delivered to your door or inbox:
Sign up